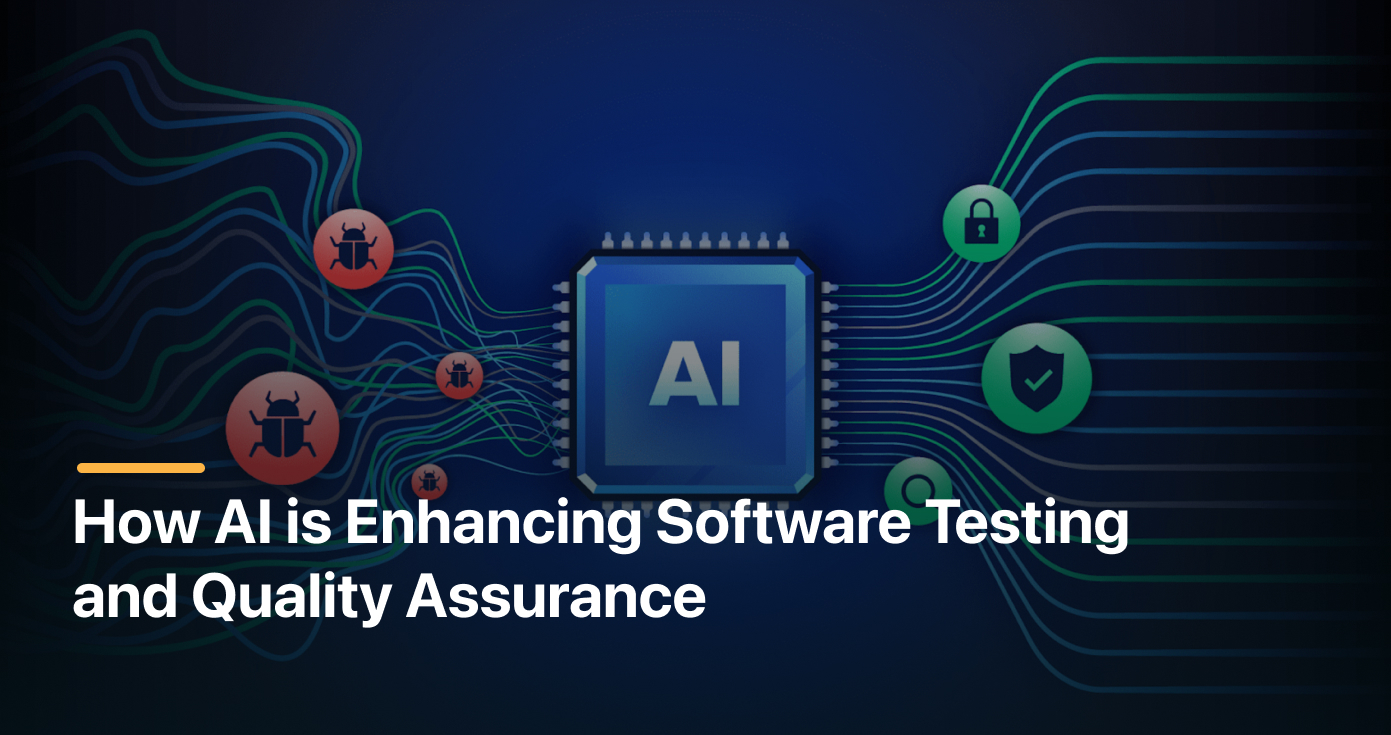
How AI is Enhancing Software Testing and Quality Assurance
In today’s fast-paced software development environment, ensuring the quality of applications is more critical than ever. Traditional methods of software testing are being complemented and, in many cases, replaced by AI-powered tools that automate and enhance the quality assurance (QA) process. AI quality assurance tools are revolutionizing the way testing is conducted, providing faster, more accurate, and more efficient results. With the rise of generative AI in quality assurance, tasks like bug detection, regression testing, and performance monitoring are becoming increasingly automated, reducing human error and improving overall software reliability.
As AI technology continues to evolve, many are asking, How advanced is AI right now in the QA field? For those looking to pursue a career in this space, understanding how to become a software quality assurance engineer or an AI quality assurance specialist is crucial. The demand for skilled professionals is on the rise, as the best AI development companies are increasingly incorporating AI-driven solutions into their QA processes, setting new standards in software testing and quality control. This article explores how AI is transforming software testing and QA, the role of generative AI, and the skills needed to succeed in this growing field.
QAs and How AI is Making the Processes of Software Testing Better:
- Automation of Repetitive Testing Tasks: AI quality assurance tools perform repetitive acts like regression tests, user interface tests, and smoke tests. This cuts down on time and saves people’s efforts to do it by themselves and thus allows teams to work on more challenging tasks.
- Faster bug detection and resolution: With AI, it is easy to parse through the code to diagnose a problem or to discover a vulnerability. Because the problems are identified at the beginning of the development cycle, it is a shorter time to test the software, and it increases the level of software quality.
- Predictive Analytics for Test Coverage: AI employs machine learning to determine the parts of an application most prone to experiencing deficiencies through past data. It makes testing more efficient because crucial areas that need to be tested are tested more rigorously.
- Improved Accuracy in Test Execution: Automating tests could prove beneficial because AI tools in particular are better at running tests than humans in that they are unlikely to make certain errors. This results in more accurate results and provides an assurance that the software application functions as intended for shipping to other channels.
- Generative AI in Quality Assurance: Generative AI is deployed in QA to generate test scripts and mimic different forms of user interactions and generate various tests. It does not only enhance test coverage but also reduces the amount of time used to develop manual test scenarios.
- Smarter Test Case Generation: Test cases can be automatically created by using AI quality assurance tools employing application code or user stories to achieve assured coverage of functional and edge conditions. This lowers the probability of early escapes of defects and enhances software reliability.
- Continuous Testing and Monitoring: Testing tools based on AI help CI/CD practices through automation of tests at all stages of SDLC. This also shows that with the use of AI, tests can be run concurrently with development so that when there are problems, they can immediately be picked.
- Real-Time Feedback for Developers: Quality assurance tools based on artificial intelligence generate detailed feedback to developers and help prevent situation escalation. This results within a shorter span of time and delivers better quality software.
- AI in Performance and Load Testing: Through AI, loads and performance testing can be conducted where numerous users are simultaneously created from the software to mimic thousands of users. It, as a result, determines the areas of limited scalability and potential problem areas in a software so that service providers realize high traffic and the performance of a software shall not suffer.
- Role of AI Quality Assurance Specialist: With the entry of artificial intelligence in QA, there is a need for an AI quality assurance specialist. AI technologists working in this area need to have knowledge and understanding of both existing AI techniques and conventional testing methodologies.
- Training AI for Better Results: AI tools are designed to progress through the particular subject field as well as get better through learning from the results of previous trials as well as from feedback from developers. This is true because with more data, the AI systems are trained to identify intricate problems and recommend changes for enhancing their performance.
- Becoming a Software Quality Assurance Engineer: Automated software testing is a new trend that has appeared in the QA industry; therefore, IT professionals who want to become software quality assurance engineers need to enhance their knowledge of both classical and AI methods. It will become critical for future careers in this field to understand how AI improves the QA processes.
- Adoption by the Best AI Development Companies: Top AI development companies are adopting an AI-based testing tool within their quality assurance arsenal. In using these pioneering approaches, they are able to deliver releases more quickly and with greater certainty—a higher-water mark for the software industry.
Artificial intelligence is gradually finding its way into software testing and quality assurance with automation, enhanced testing precision, and real-time feedback. When such advanced tools as generative AI are being developed, constant employment of AI quality assurance specialists, as well as the deployment of AI-driven testing solutions, will be the major factors for guaranteeing the delivery of quality software.
Read More: Why AI is Revolutionizing Software Development: Benefits and Challenges
Conclusion
In conclusion, it can be said that AI is changing the software testing and quality assurance (QA) industry quite significantly. AI quality assurance tools have made it possible to automate recurrent test processes, identify faults more quickly, and create a better case, thereby increasing both productivity and quality.
The advancements in generative AI in quality assurance have gone further in improving testing by the auto-generation of tapes and testing of user endeavors. But as technology advances, the question arises: how advanced AI is right now in the field of QA, thanks to new applications that increase the efficiency of processes that were impossible before. If people are interested in joining this field, they should learn how to become a software quality assurance engineer or an AI QA specialist, as the need for a specialist in AI-based testing is rapidly increasing.
The top AI development companies already incorporate these sophisticated AI solutions into quality assurance, creating new standards for testing software. Finally, I want to emphasize that AI is not only revolutionizing quality assurance in terms of speed and accuracy but the overall approach to software testing is becoming smarter, way more efficient, and easily scalable at that.